5. FAQ (MANUFACIA Operation)
5.1. Error occurs to upload data
Case1: CSV file (time-series/vibration)
CSV file should satisfy the required specification. For detail, see Users manual -> Supported data formats: Time-series data, vibration data.
Case2: Image files
Image file should be the format as defined in Users manual -> Supported data formats: Image data.
5.2. Training fails
For the models whose training failed will be shown in the image below and there is no model detail to display.
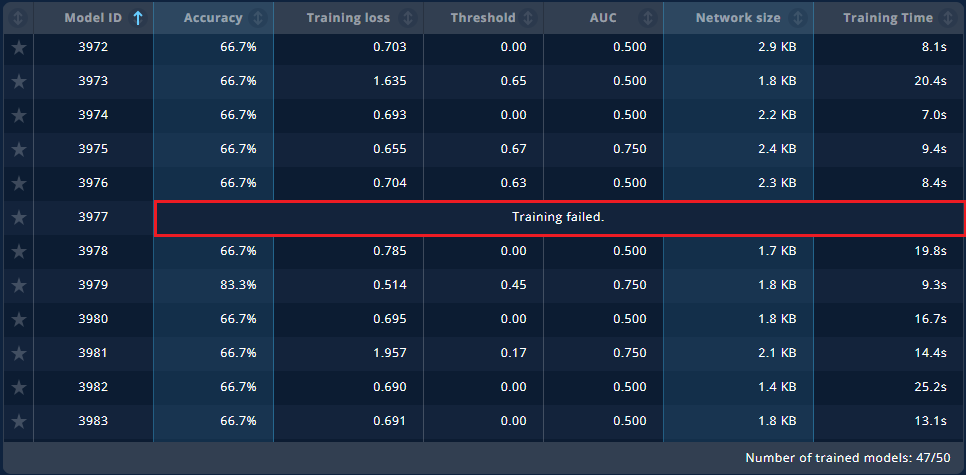
Here are possible reasons of training error.
Inconsistent tag to assets to be uploaded and label to assets
Refer to Example of tag settings, Label assets and the following Label examples to see if there is any inconsistency in the setting.
Batch size is too big for the number of uploaded assets
Refer to Batch to see the relation of the number of assets, batch size and iteration.
Run out of resource
Depending on the data using or the model creating, it may happen that resource runs out on the server where MANUFACIA runs and then the training fails. If it is the case, add more memory may solve the problem. Especially for image data, if the default image size after preprocessing is much bigger than default size of 224x224, it may run short of memory to create a model by ResNet50 and then the training fails.
Note
See Trouble Shooting -> Local disk is full. Which files may I delete? to know how to check free disk space.
Training datasets, training preprocessing setting’s problem
See next section Low AI model accuracy.
5.3. Adding assets takes time
There is no limit to upload data files, but it takes time as data size increases. Do not shut down the PC while uploading.
Especially, image data files may have more volume and number of files tends to be bigger, it will slow down the process. To keep a nice balance between accuracy and performance, there is an upper limit of 512 x 512 for the image size. Cropping or scaling down big image data files in advance will shorten the upload duration. Also, a zipped file can make the process faster.
5.4. Low AI model accuracy
If the AI model you created is less accurate, you can consider the following reasons.
Too little training data
The accuracy will be lower, if training data is little, especially when many parameters are necessary for training. Training the model with many parameters and with less data, it could be just overfitting even if the result may seem good. In such the case, increase the amount of data or number of classes could improve accuracy.
Please use 100 to 300 dataset files per label as a guide. For unsupervised learning, it is not always the case for anomalous validation datasets.
Datasets balance is bad for each label
If there is a huge difference of datasets between labels for training and validation datasets, training result will be more affected by the label with higher number of datasets and will not be practical even if the accuracy is high. In such the case, try to prepare balanced datasets per label, then the training will properly proceed. For image datasets, data augmentation tool can balance the amount of datasets per label.
Training/validation datasets label is not correct, or wrong tags are applied to label assets.
Wrong labeling to datasets, incorrect separation of datasets to folders, wrong labeling to properly prepared assets can cause low model accuracy. For anomaly detection, supervised or unsupervised learning, Histogram is available after the training to see if normal and anomaly labels are not mixed together.
Datasets used for the training do not represent validation datasets deviation
Not only while training but also while using the AI model on the edge device to infer the sample, if it is normal or anomalous, training datasets should properly represent distribution of labels: normal data for positive, anomalous data for negative. If they don’t, created AI cannot correctly predict with new data as mentioned in Users manual -> How to evaluate AI judgement.
For image datasets, data augmentation tool which is offered separately from MANUFACIA can augment datasets for training by changing several graphic characteristics within a certain range. By completing training datasets distribution with augmented datasets, threshold between normal and anomaly will be more clear and apparent, which will help improving model accuracy.
For time-series or vibration datasets, there is no augmentation possibility available such as for image datasets.
If it is hard to gather enough datasets for training, retraining AI model can improve the accuracy while taking data during operation.
Setting to read the data is not optimal (Preprocess setting)
For time-series or vibration datasets, selection of the columns to read can be not optimal and is missing a column which is decisive to the sample’s being normal or anomalous. The data such as ID, line number or time step are not really necessary for the training, remember to switch off those columns.
Preprocessing is not optimal
The accuracy can be improved by customizing preset preprocessing depending on the data type.
For time-series or vibration datasets, for example, to switch on interpolate option, or change normalize/standardize setting, for image datasets to crop the image in the center where the interesting part is or raise image size may improve accuracy. Try together with data augmentation tool mentioned above.
For vibration datasets, in addition to time-series datasets, sliding windows setting may be not optimal and has a low accuracy.
To repeat changing setting and training, it will take time if, for time-series datasets files are long (e.g. more than 5000 lines) or the number of data files are many (e.g. more than 1000). In those cases, it will be effective to thinner the CSV files with the help of CSV format checker for time-series datasets, and resize the images smaller for image datasets, to reduce training time and see how the preprocessing setting change will affect the training result.
See Users manual -> Define pre-processing (Time-series/vibration data), or Users manual -> Define pre-processing (Image data) depending on the datasets you are using.
Number of models are too small
Hyper parameters that cannot be set in the preprocessing UI such as the number of epochs or learning rate will be randomly set. Therefore the number of models created is to some extent important to create models with high accuracy. It is recommended to confirm first, if the training will go through without problem with small number of models to create such as 10 to 20.
To improve the accuracy or if many models failed to train, try to find better setting by changing preprocessing setting little by little (only one parameter at the same time) mentioned below, then increase the number of models to create. (50 to 100)
If the accuracy will not improve or MCC will not be closer to 1.0 by creating more models, it is possible that the number of datasets files are too little, or labeling to the datasets is partly wrong and some samples are with the wrong label. Of course, the datasets are still hard to handle by MANUFACIA, this possibility is there, too.
If trainings of all models fail, then it might be because of the input file format. If using CSV files, use CSV format checker to find and solve the problem.
5.5. No idea which AI model is good
In general, a model whose accuracy is high and training loss is low is told to be good. However, number of dataset files for training or distribution of datasets for a same label can also affect if the model can be good or bad.
Matthews correlation coefficient (MCC) which was introduced in v2.2 can properly evaluate models for the cases in which the accuracy is high but the model is rather useless.
Criteria how to identify good models are followings.
MCC(Matthews Correlation Coefficient)is close to 1.0.
AUC is close to 1.0.
Validation datasets samples are clearly separated. (In the histogram distributions of labels do not overlap. Available for anomaly detection only.)
Low training loss value. (Training loss curve converges to zero as training proceeds.)
The one with smaller network size, if all criteria above are fulfilled.
For anomaly detection, threshold between “normal” and “anomaly” can be adjusted after model creation. For example, to improve inference of anomaly data, decrease threshold will help increase detection percentage of anomaly data. (There are cases that it cannot be changed due to datasets.)
Remove models that did not train properly. The following cases show the model whose training was not successful.
No accuracy, no training loss value are displayed.
MCC is below 0.5 zero. (It is worse than random label prediction)
Threshold or distance is zero.
Training loss curve does not converge to zero.
SmoothGrad is just black. (For supervised learning only)
5.6. Cannot pause the training
See Users manual -> Train models for how the pause function works in MANUFACIA.
5.7. Recreate AI model by adding new data
If you want to additionally consider new datasets with an existing AI model, a new project should be created to generate new AI models.
Add data in the lab where you want to create a new model (See Register assets), then create a new project (See Create a new project) to make new AI models.
5.8. Want to change the tag assigned to assets
Once a tag assigned to assets and then the assets have been registered (uploaded), it cannot be modified. Create a new lab and then set tags of the wish to the assets.
5.9. Want to delete lab
To delete a lab, the projects registered in the lab should been deleted in advance. With assets registered in the lab, it can be deleted.
To delete a lab, click the three vertical dots in the upper right of the lab and select [Delete].
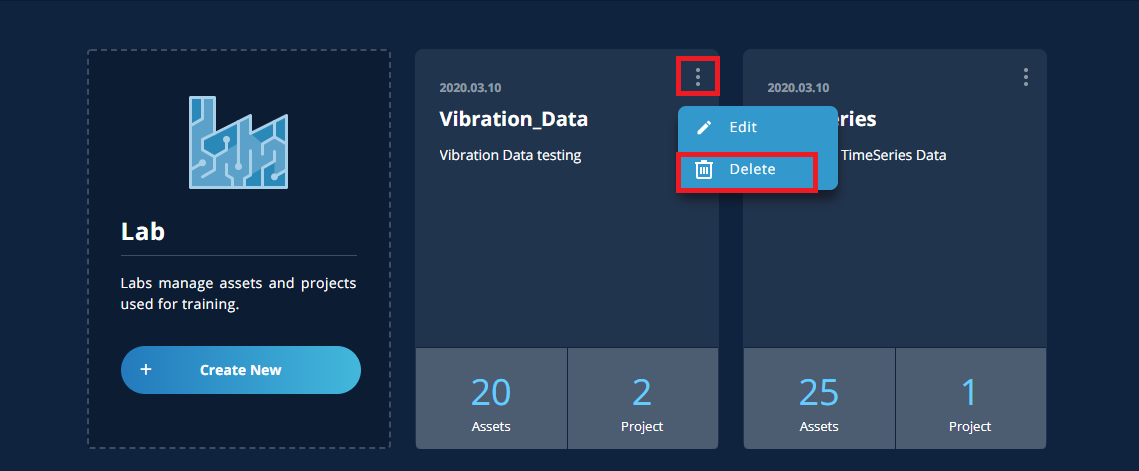
5.10. Want to delete projects
If there is any other project which was created by [Use existing model] associated with the project to be deleted, delete the associated project first and then the project to be deleted.
To delete a project, click the three vertical dots in the upper right corner of the project and select [Delete].
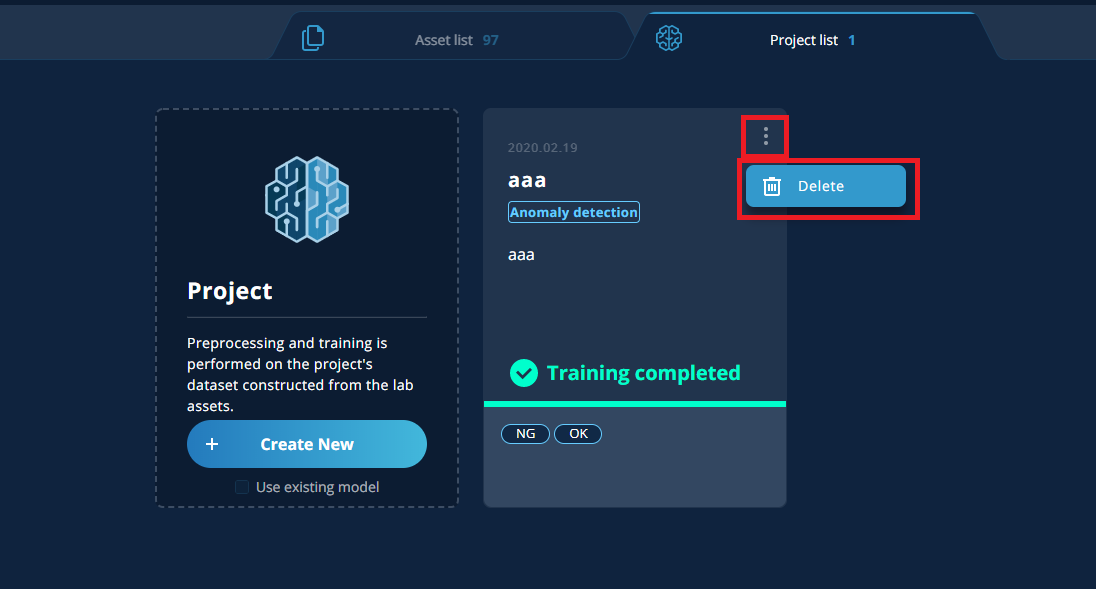
5.11. Want to delete assets
Currently, assets cannot be deleted.
5.12. Models will not be plotted in the scatter diagram
In some cases, models are plotted in the scatter diagram. Since the training error set 100 to the threshold of maximum value, models with the training error above the threshold will not be displayed or N/A.
5.13. Labels cannot be added
Labels can be registered up to 20. If there are already 20, [+ Label Creation] will not be displayed.