1. Before starting MANUFACIA
1.1. Lexicon
Account
12 accounts are available to create labs inside. There is no access restriction with accounts.
Lab
Place to collect data and training results for training and prediction.
Asset
File used for training and prediction.
Project
Place where AI modules are created. Preprocessing and training using data sets created with asset.
Class
Class refers to the group to classify.
Label
Name of the class. In case of anomaly detection, labels ‘normal’ and ‘anomaly’ are prepared. For multi-class classification, set labels according to the number of classes.
Tag
The name of the group of assets handled by MANUFACIA (For more information, see Set tags).
Model/AI model
Definition of neural network and data processing that are created by training.
Deploy
To send the model to operation environment such as edge device.
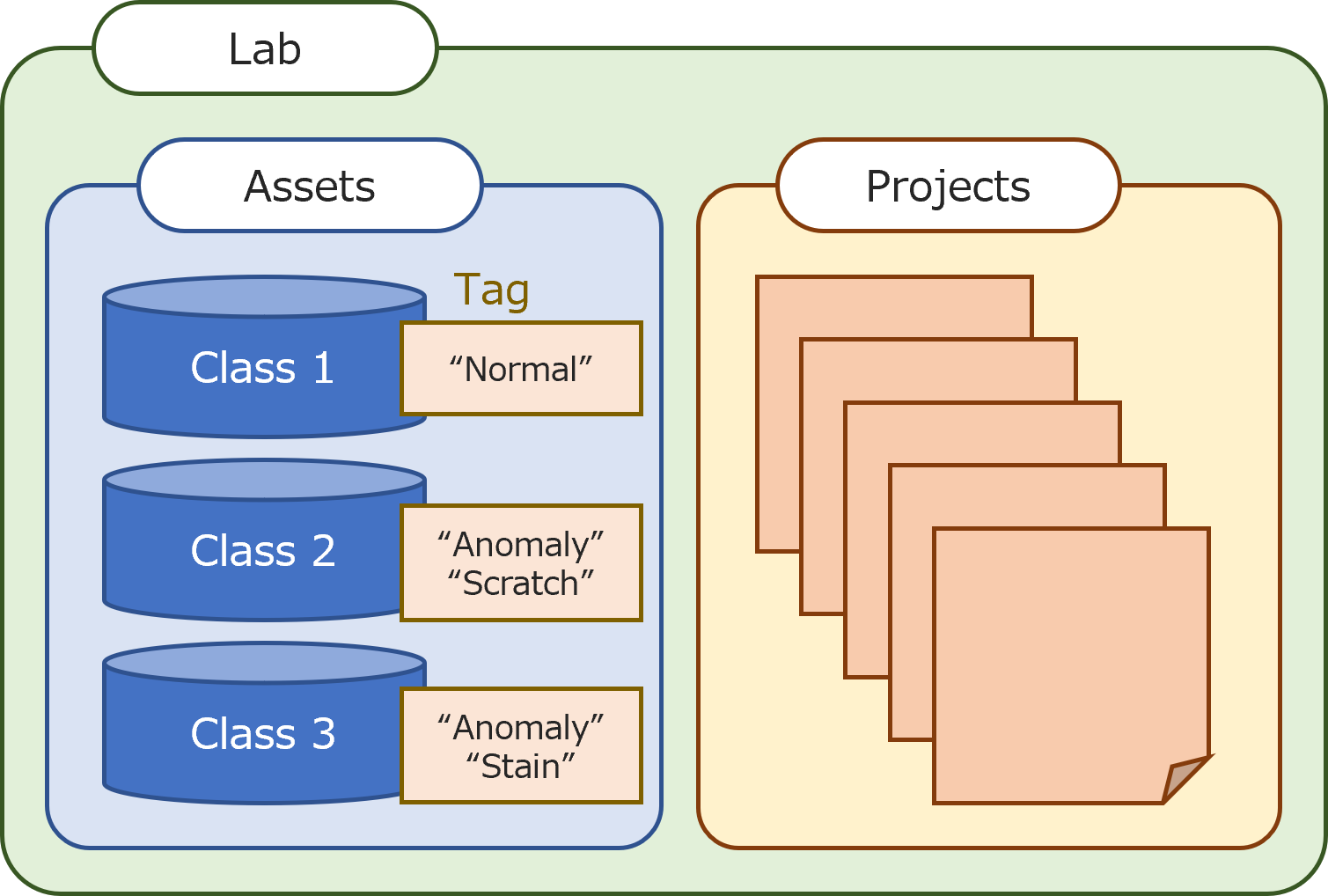
1.2. What MANUFACIA can do
MANUFACIA learns feature of classes from correctly labeled assets, then generates neural network models. (AI model) By implementing the AI model to devices or instruments, it can help deal with the tasks that have been relied on the intuition of experienced workers or their eyes.
1.2.1. Supported data formats
1.2.1.1. Time-series data, vibration data
File format: .CSV
Detail specification:
Items |
Specifications |
---|---|
First row |
The first row must be the name of each column (label name) |
2nd and subsequent rows |
There are data the rows corresponding to the label name in the first row. |
Format of each column |
The format of each column is consistent and there should be no data loss. |
Delimiter |
The delimiter is a comma ‘ , ‘. |
Character code |
The character code is UTF-8. (with BOM. CSV files from Excel are with BOM.) |
File unit |
Divide different classes (normal/anomaly) in separate csv files. |
Note
Vibration data extracts frequency components.
Tip
For CSV data, use the CSV format checker to know if the format is supported by MANUFACIA. This tool can also process data files in several ways. For further information, refer to CSV format checker user manual.
1.2.1.2. Image data
File format: .BMP .PNG .JPG
Hint
JPG format is a lossy compression, it is recommended to use .BMP or .PNG instead to improve the model accuracy.
Lighting condition at the image shooting area also affects the model quality. If the focus area is just a part of the image, or if it is necessary to tile images from a big one, data augmentation tool can be used, which can also augment image data files or retouch them. For the further information, refer to Image data augmentation tool user manual.
1.2.2. AI model generated by MANUFACIA
1.2.2.1. AI model types
With MANUFACIA, three different kinds of AI model from two different data types (csv file, image file) can be generated. The description of these three types is as follows.
Anomaly detection, supervised learning
A model will be generated for anomaly detection. Using both “normal” and “anomaly” data for training, it is called supervised learning. The threshold of generated model can be adjusted after training. Using this model, inference will tell which of the labels of “normal” and “anomaly” the input data is close to.
Multi-class classification (supervised learning)
A model will be generated from more than two labels of datasets for classification. The threshold of generated model cannot be modified. Using this model, inference will tell the probability of the input data to correspond to each label used for model generation.
Anomaly detection, unsupervised learning
A model will be generated for anomaly detection. Using only “normal” data* for training, it is called unsupervised learning. The distance (range of being “normal”) of generated model can be adjusted after training. Using this model, inference will tell how far the input data is from being “normal”. This approach is suitable for cases with little “anomaly” data.
Note
Note For validation, “anomaly” data is necessary.
1.2.2.2. AI model selection
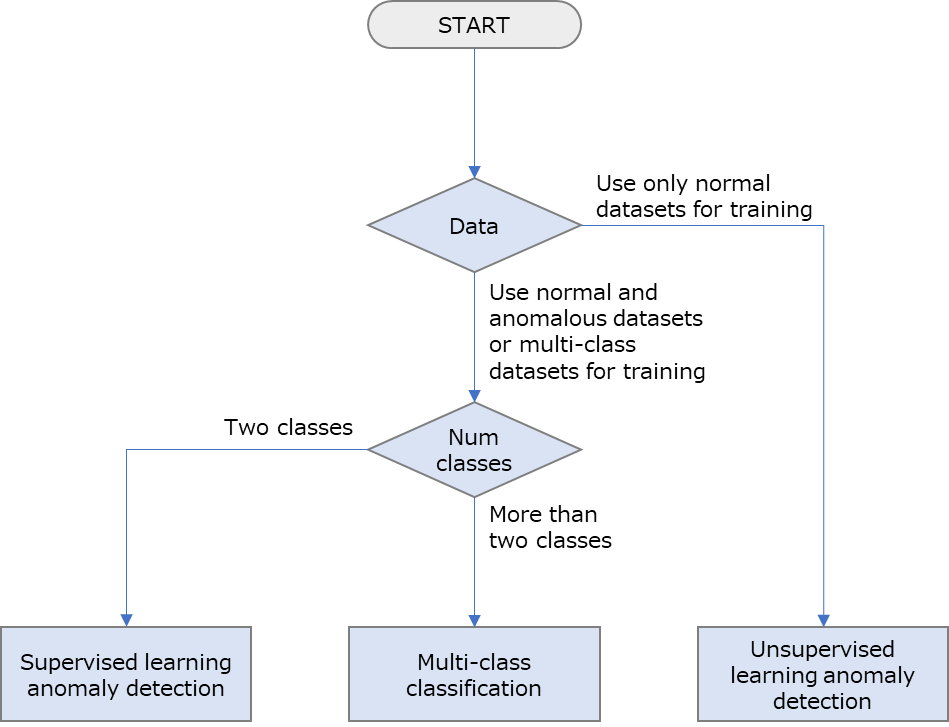
Important
To use two labels for datasets (e.g. “normal” and “anomaly”), use “Anomaly detection, supervised learning”.
1.2.3. Turn the generated AI models to account
AI models generated by MANUFACIA can be deployed to edge devices, some tools are also offered to users to manipulate the AI models, such as to infer with an input data, or to request results and so on, and a system development is necessary to fit to the local operation. Greenia Embedded SDK(Software Development Kit for C)and Python API (Application Programming Interface) are available.
1.3. How to evaluate AI judgement
In anomaly detection (binary classification), labels of “Positive” / “Negative” will be often used, which do not necessarily match our natural sense. For the issues of breakage of facility or machines at production site which MANUFACIA is supposed to solve, we would think that it means something positive and normal if there is no breakage, and negative and anomalous if there is breakage. However, in AI cases it might be contrary; the purpose of using AI is to find breakage as early as possible, then the situation to have found breakage is positive, and if there is nothing happening then it is negative. In this case, positive cases are very rare to happen than the negative cases.
For the judgement if it is positive or negative, it does not mean that everything which was judged as positive/negative is exactly the same. There should be a tolerance to some extent to be judged as positive/negative. If the distribution of two labels will not be cleanly separated, then there will be a problem.
Let us think about an inspection process to find product defects. The distribution of “ok” products (blue) and “ng” products (red) somehow overlap. If we do not want to allow ANY defect to avoid market claims and draw the threshold as below, then it means everything on the left is “ok” and on the right is “ng”. There is nothing wrong with the product which AI judges as “ok”, but the area in light blue which should also be “ok” should be treated by AI “ng”. To save these hidden “ok”s, we will need extra inspection which is actually redundant.
In general cases, there should be cases;
predicted negative although it is positive (light blue area).
predicted positive although it is negative (pink area).
This threshold should be properly adjusted due to situation.
Before that, it is very important to prepare datasets for training that two cases are clearly separated to reduce this overlap as much as possible. It will be difficult to expect high performance to the AI, if datasets are too small or they do not correctly represent the real distribution, and without saying, if labeling of the training datasets was not correctly done.
1.4. Start MANUFACIA
MANUFACIA is a Web application, starts service at server side, users will access to the server from client PC via browser. Please refer to the Installation Manual -> Install for the information how to start service on server.
Start the browser on client PC, and access the URL given below. In {IP_ADDRESS}, enter the IP address you found when installing the application.
http://{IP_ADDRESS}:3200/
If the following window appears, the installed application is running.
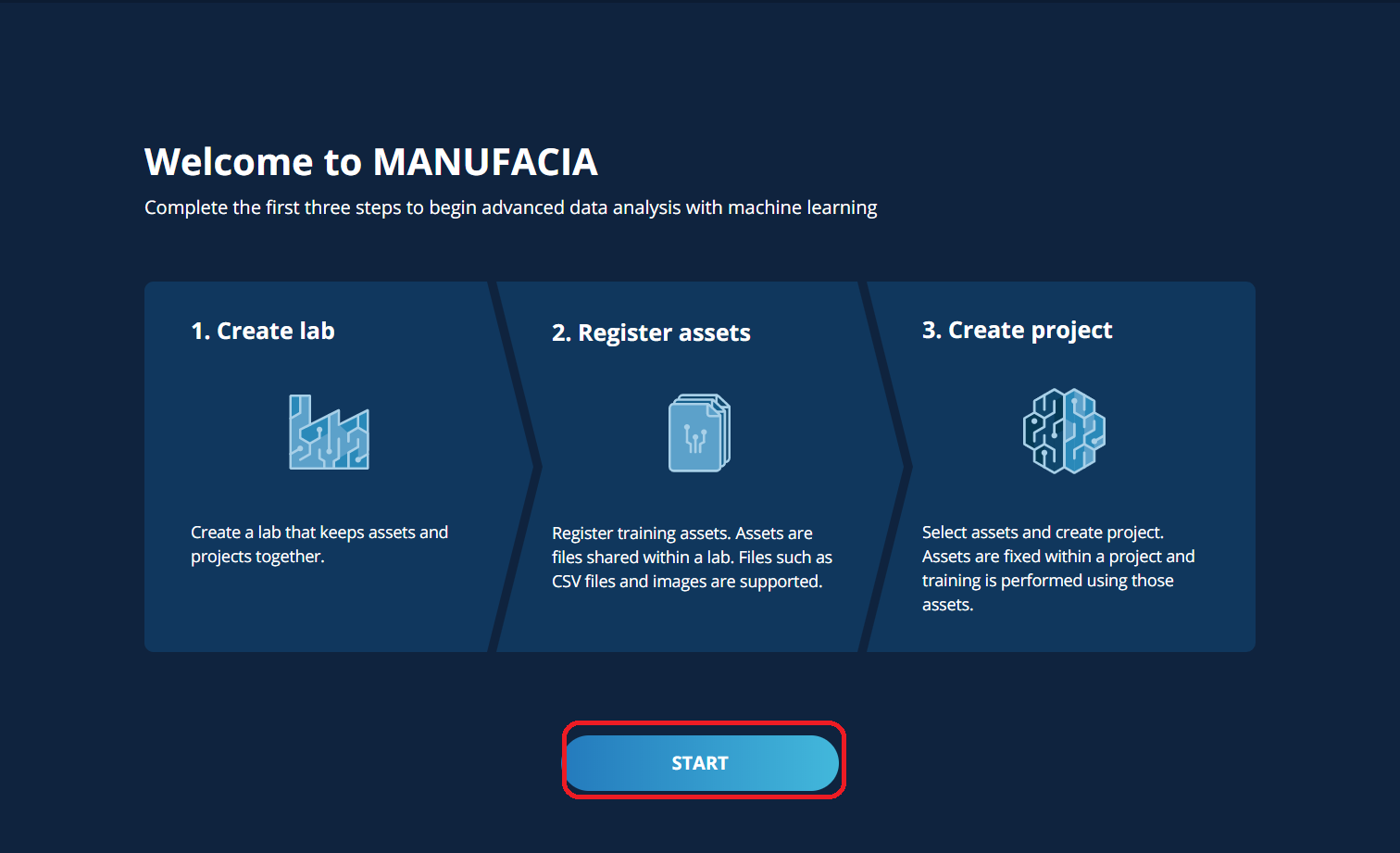
1.4.1. Recommended hardware specification
For specification of GPU servers or client PC, refer to FAQ (General) -> Recommended hardware specification.
1.4.2. Display language
Switching browser’s display language, MANUFACIA UI will be displayed in Japanese/English/Korean/Chinese_CN/Chinese_TW. If the browser language setting is none of these languages, it will be displayed in English.